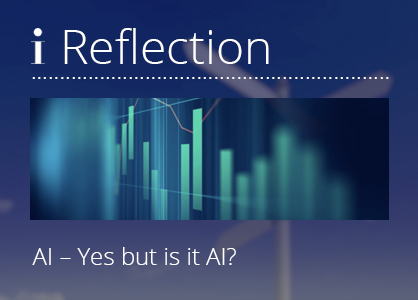
AI! Yes, but…. is it AI we are talking about?
AI (Artificial Intelligence), AI (Augmented Intelligence), ML (Machine Learning), RPA (Robotic Process Automation) and other more or less well-known acronyms are popping up everywhere, promising ever brighter futures, or futures resembling, Huxley-like, Brave new worlds. The truth? Long term, on average, probably somewhere in the middle (as usual). The problem however is that as long as the “debate” focus on the extremes of the usage spectrum is it hard to a agree on reasonable basis for AI investments.
To start with, lets agree that AI (Artificial Intelligence) can do absolute wonders, both financially and logistically, by analyzing and predicting patterns in how a city dispensing-machine planning should unfold or a major clothing company shelf usage tactic should be set-up. Even in more advanced applications such as making primary evaluations of CV’s and personal letters for recruiters, it can do magic. One example is the solution by a company doing really well in that field i.e. Layke Analytics. Another fantastic example of what AI can contribute to is the Ericsson/UNICEF school connectivity initiative that can be viewed in this LinkedIn post. Still, however value contributing, these information algorithms are based on preset patterns with very targeted issues at hand that are being supported.
Let’s also agree that AI will not take over the world, it will not replace the classic scenes of humanity such as philosophy, religion, music, interpersonal relationships etc. AI computers will not become humans. For instance, Layke’s solutions do not do the hiring and interviewing but it helps tremendously in providing a fitting cross segment of thousands of applicants that human recruiters subsequently will meet with and interview, i.e. a schoolbook example of AI (Augmented Intelligence).
Why is all this important? Because we need to know how far we can plan for, and count on, AI (both of them) in what could, for the purpose of this reflection, be labeled strategic management. Too little AI will eventually render the company lagging its competitors and an over belief in AI may rapidly send it one-way over a cliff into the deep gorge of bankruptcy, or worse.
A quick review of what some significant thinkers in the field have had to say in recent years reveals somewhat of a consensus.
In 2018, Joshua Sokol wrote in Quanta Magazine Why Artificial Intelligence Like AlphaZero Has Trouble With the Real World … researchers are struggling to apply these systems beyond the arcade. Sokol focuses on the AI challenge of gaming but do relate that to some “real world” challenges.
Some quotes from Sokols’s article:
- Of course, the companies investing money in these and similar systems have grander ambitions than just dominating video-game tournaments. Research teams like DeepMind hope to apply similar methods to real-world problems like building room-temperature superconductors, or understanding the origami needed to fold proteins into potent drug molecules. And of course, many practitioners hope to eventually build up to artificial general intelligence, an ill-defined but captivating goal in which a machine could think like a person, with the versatility to attack many different kinds of problems.
- One characteristic shared by many games, chess and Go included, is that players can see all the pieces on both sides at all times. Each player always has what’s termed “perfect information” about the state of the game. However devilishly complex the game gets, all you need to do is think forward from the current situation. Plenty of real situations aren’t like that. Imagine asking a computer to diagnose an illness or conduct a business negotiation. “Most real-world strategic interactions involve hidden information,” said Noam Brown, a doctoral student in computer science at Carnegie Mellon University. “I feel like that’s been neglected by the majority of the AI community.”
- Many other researchers, conscious of the hype that surrounds their field, offer their own qualifiers. “I would be careful not to overestimate the significance of playing these games, for AI or jobs in general. Humans are not very good at games,” said François Chollet, a deep-learning researcher at Google. “But keep in mind that very simple, specialized tools can actually achieve a lot,” he said.
In his 2019 article AI in the workplace, Julian Birkinshaw, Professor of Strategy and Entrepreneurship at the London Business School, asks What kinds of jobs will humans be left with once AI reaches its potential?
Some quotes from Birkinshaw’s article:
- Let’s be clear about what AI can and cannot do. Its “intelligence” is essentially an ability to process and build upon what has gone before. Machines are literally “trained” by exposing them to huge bodies of data –text, pictures, codified speech—that allow them to spot patterns and make predictions. This can lead to seemingly-creative outcomes but it’s a form of creativity that is confined within a narrow set of boundaries: it is about drawing an inference from past experience.
- To win the race, it [AI] needs to make decisions about which customers to target and what new products or services might be devised to attract them. Here, AI’s limitations are revealed. Decisions such as these require intuition, imagination – and, crucially, an ability to pull together items of information from many different sources. Lateral thinking involves far more than computing power, however vast. No computer ever dreamed up a cool new brand.
- Facebook’s systems could not see the threats. It’s not simply that AI failed to spot the elephant in the room; AI was in a completely different room.
In summary he concludes that:
- Computers have enormous potential but they are only able to process what has gone before
- AI can provide essential support to a company but they will never differentiate it from competitors
- AI will help people focus on the valuable creative and intuitive parts of their jobs.
In a 2020 webinar, Jesper Martell, co-founder and CEO of Comintelli, vendor of AI-based software Intelligence2day® concluded that:
- AI/ML does complement and help intelligence analysts by very quickly analyzing very large amounts of texts and detecting patterns and topis, BUT does not ask the strategic questions, make decisions or take action.
- AI/ML does solve some challenges when it comes to automatically classifying and organizing large volumes of content BUT the challenge is then moved to getting better content. Content needs tp be of higher quality and preferably in full text for AI/ML to give good results.
- AI/ML works best with very large amounts of articles and interactve users (eg on internet Amazon, Twitter, Google) BUT in the enterprise world, even the largest organizations are small from an AI perspective.
Finally, in its Technology Quarterly section, June 11th, 2020, the premium magazine The Economist dedicated a series of articles to AI and just the titles of them are enough to get the gist:
- An understanding of AI’s limitations is starting to sink in – After years of hype, many people feel AI has failed to deliver, says Tim Cross.
- Businesses are finding AI hard to adopt – Not every company is an internet giant.
- The cost of training machines is becoming a problem – Increased complexity and competition are part of it.
- Humans will add to AI’s limitations – It will slow progress even more, but another AI winter is unlikely.
- For AI, data are harder to come by than you think – When in doubt, roll your own.
The problem with all of the above is that it remains in the eyes of the beholder. Readers of this text (that have made it through so far) will either be furious that this is yet one more “AI-is-a-flaw-article” and close the browser and AI-sceptics may relax and say “I told you so! No worries”. Both categories, please read again, from the start! Also, if in doubt, read the linked source texts. There is a reason for why those in particular have been chosen; They are balanced.
No doubt, AI is here to stay! It will drive significant changes in organization theory and applied management and well catered for it will be an enormous support.
Birkinshaw again: “As more and more information becomes available to an ever-expanding cohort of individuals in a firm, the role of managers will have to evolve.” ….. “An ability to evaluate the output of AI, creativity, imagination, drawing strands of inspiration from disparate sources and a willingness to challenge orthodoxy – these are the capabilities organisations need to develop in a world where AI becomes increasingly widespread. But no less important will be the manager’s efforts to encourage colleagues to give expression to these quintessentially human talents.”
For pure process and logistics optimization and automation, deployment of AI applications is thus a “no brainer” (not referring to the technical complexity though), but what about management and strategy? For all we have seen from AI to date, it is clear that unless some completely unforeseen development evolve, humans will remain in charge, cater for innovation, decisions and actions as well all people-to-people interactions. All this brings us to the difference between Artificial Intelligence and Augmented Intelligence, the latter being the concept that already today can make a huge difference in strategic management quality and efficiency but we need to agree on what we are talking about.
Artificial Intelligence has since long (it all started in the 1950ies) evolved into one of the overly hyped terms that means all and nothing. A smart machine uses AI, a search engine with pattern matching uses AI, self-driving cars use AI and so forth. It is truly an acronym with no real meaning today other than the hype factor. Better then to revert to one of the more technical terms underneath AI, namely Machine Learning, i.e. the term for a type of program that can adjust its own algorithms based on evolving “experiences”. Machine Learning is also a key component of Augmented Intelligence, i.e. programs and applications that enhances the intellectual efficiency of humans. The Layke case above is a perfect example and the “experience loop” back into the program is simple; “these are the candidates we have chosen” and the program will continue to refinance its algorithms for identifying the best candidates for an interview accordingly.
Augmented Intelligence can also operate without machine learning as long as it has sufficient pattern matching and context recognizing abilities such as preprogrammed algorithms for autoclassification of text-based content. The ideal Augmented Intelligence platform make use of both in a balanced mix depending on the decision support that is targeted.
Bringing these thoughts into the domains of Insights Management makes it apparent that the question of “What?” is absolutely crucial to render any sort of return on investment in these fields of technology. What discipline is to be supported? What types of decisions are to be enhanced? What shall we do with the results? The answers to these, and often many more, questions form the basis for case by case developed information models that should resemble the different organizations operations and strategy, i.e. information models that can define the ML algorithm training loops as well as the manually configurable pattern recognizing algorithms needed to deliver the value sought for.
Inzyon Reflections is a series of brief thoughts and observations with a bearing on insights management in general and, every now and then, sustainability matters in particular. For additional Inzyon Reflections, see here.